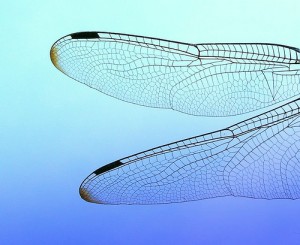
Every good researcher knows what a vital role data sharing plays in the data life cycle. So in a perfect world, the decision to share data would be an easy one to make. By effortlessly passing data on to someone else, you would receive all the spectacular rewards data sharing has to offer – a reputation boost for yourself and a colleague, the satisfaction of fueling new projects and discoveries, and enough leisure time left over to start a new book or take up bird watching. What more could a researcher ask for?
Not surprisingly, however, researchers often find themselves at the mercy of the same mess of hierarchies, rules, and complications that plague the rest of the professional world. In this data story, a novice researcher finds herself in the murkier depths of data sharing where the boundaries of academic territory can seem hazy.
Danielle had just started out in ecological research, but already found herself invited to participate in a working group on species interactions. The group was made up of many experienced scientists in the midst of their careers… and Danielle. But what Danielle lacked in experience, she made up for in connections. The working group was very interested in a dataset Danielle had been working on for several years and had hopeful plans of incorporating it into a model they were developing. The problem? While Danielle held a copy of the dataset, she did not actually own it. In her work with faculty mentor Dr. Stevenson, Danielle had enjoyed liberal access to the PI’s impressive dataset. With a few years of collaborative work behind them, mentor and mentee had developed a trusting relationship where Danielle received an uncommon level of data access.
As someone already involved with the dataset, Danielle was in an ideal position to act as information broker between the enthusiastic working group and her mentor. With these connections, Danielle expected the data sharing process to be a smooth one. However, uneager to upset Stevenson or jeopardize their work relationship, Danielle knew she would first need to gain permission to share the data.
But when Danielle approached Stevenson with the request, she discovered the situation was more complicated than she had anticipated. Dr. Stevenson wanted to help Danielle and give her the information her working group needed, but his data was undergoing revisions. Advances in species identification with DNA barcoding had made it possible to more accurately distinguish one species from another. But with the shift away from morphological characteristics identification, the species in Stevenson’s dataset were suddenly thrown into question. After years of meticulously collecting and maintaining the data, the last thing Stevenson wanted was for it to become obsolescent. Determined to present a perfectly accurate dataset that would maintain relevance in a changing world, he embraced the new technology and began applying it to his existing samples. Revising the large body of data was possible, but it would take time. Meanwhile, Stevenson was hesitant to share the taxonomic databases while they underwent revisions, lest they later be found inaccurate. When those revisions would be completed was anyone’s guess.
Caught between her allegiance to the working group and her respect for Stevenson, Danielle found herself in the unenviable role of middle man. Emails zinged back and forth: polite pleas from the working group, and apologetically resolute responses from Stevenson. After months of correspondence and bouncing between working group and mentor, Danielle’s efforts have yet to pay off. The dataset remains in the senior PI’s hands (in, Danielle hopes, a final draft version!).
While the uncertain outcome may remain a hurdle for the working group, Danielle feels assured she has made the right decision in waiting for Stevenson’s revised dataset. She has done what she can to push the project along without overstepping boundaries or straining her relationship with her mentor. The wait may be a temporary setback, but Danielle and the working group can rest easy knowing that when the dataset is finally delivered, its improved precision will make it all the more valuable.